The concept of face recognition is not new, nor is its implementation. The evolution of face recognition is fascinating and using computers to recognize faces has been dated back to the 1960s.
Yes, that’s correct, I said the 1960s.
From 1964 to 1966 Woodrow W. Bledsoe, along with Helen Chan and Charles Bisson of Panoramic Research, Palo Alto, California, researched programming computers to recognize human faces (Bledsoe 1966a, 1966b; Bledsoe and Chan 1965).
Since then, face recognition has gone through many evolutions. In the early 1990s, holistic approaches dominated the facial recognition community. During this period, low-dimensional features of facial images were derived using the EigenFace approach.
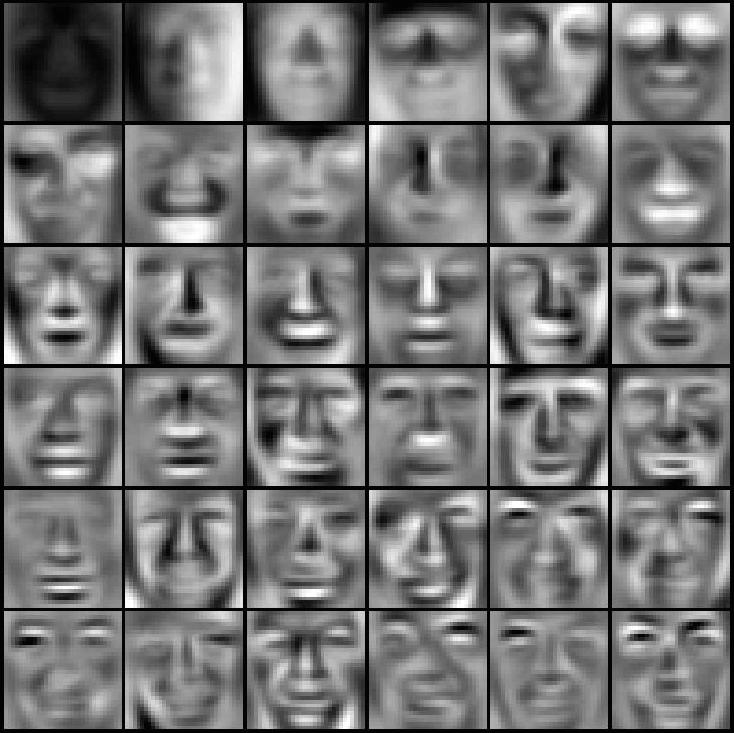
In the early 2000s, local-feature-based face recognition was introduced where discriminate features were extracted using handcrafted filters such as Gabor and LBP.
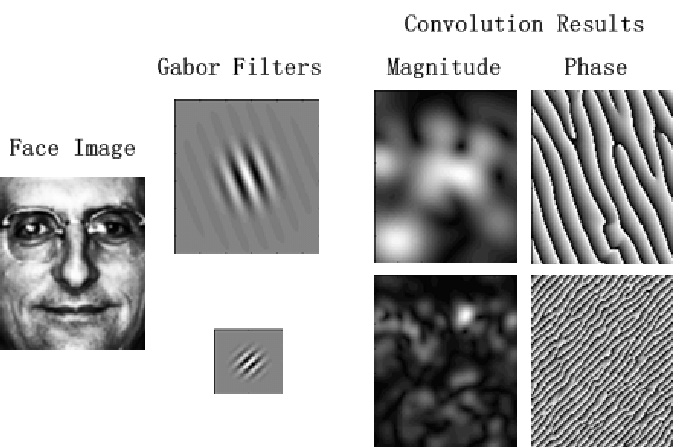
In the early 2010s, learning-based local descriptors were introduced in which local filters and encoders were learned.
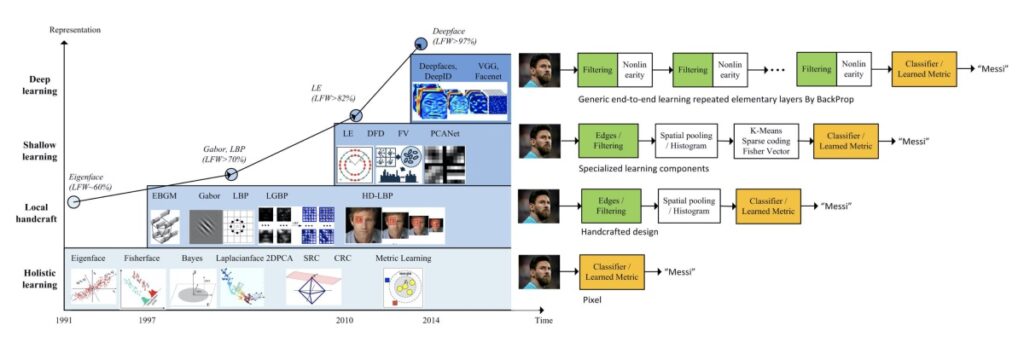
The year 2014 was marked as an important year in the evolution of facial recognition as it reshaped the research landscape of this technology. It was the year when Facebook’s DeepFace model’s accuracy (97.35%) on the LFW benchmark dataset approached human performance (97.53%) for the first time. Just three years after this breakthrough, the accuracy of face recognition reached 99.80%.
So, what changed in all these years?
All approaches up until 2014 used one- or two-layer representations such as filtering, histogram of feature codes, or distribution of the dictionary atoms to recognize the human face.
Deep learning-based models, however, used a cascade of multiple layers for feature extraction and transformation. The lower layers learn low-level features similar to Gabor and SIFT whereas the higher layers learn higher-level abstractions. That means, in the current evolution of facial recognition, what different face recognition approaches could do individually back then, can now be done using just one deep-learning-based approach.
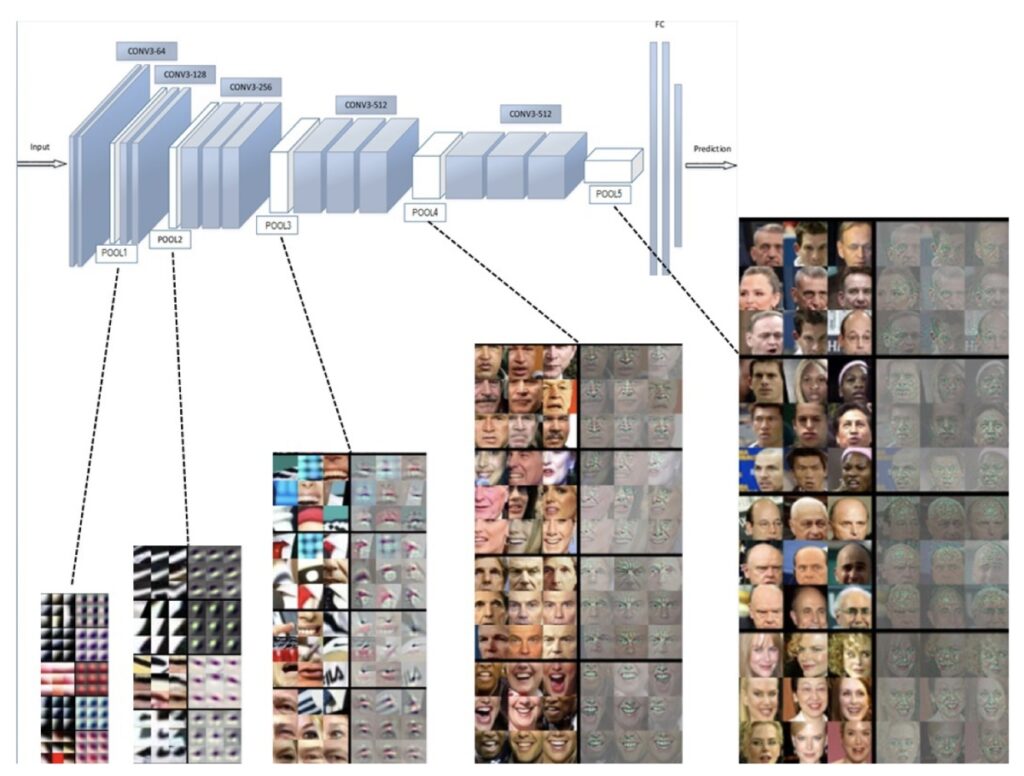