What is Edge AI, and how does it work?
Edge AI (Edge artificial intelligence) is a paradigm for crafting AI workflows that span from centralized data centers (the cloud) to the very edge of a network. The edge of a network refers to endpoints, which can even include user devices.
Edge AI stands in contrast to the more common practice where AI applications are developed and run entirely in the cloud. This is a practice that people have begun to call cloud AI.
Edge AI, on the other hand, combines Artificial Intelligence and Edge computing. Edge computing brings computation and data storage as close to the point of request as possible to deliver low latency and save bandwidth, among other advantages. AI is a wide-ranging branch of computer science concerned with building smart machines capable of performing tasks that typically require human intelligence.
With Edge AI, machine learning algorithms can run directly at the Edge, and data and information processing can happen directly onboard IoT devices, rather than in a central cloud computing facility or private data center.
Machine learning (ML) is a field of inquiry devoted to understanding and building methods that enable machines to imitate intelligent human behavior. It performs complex tasks and is a subfield of artificial intelligence.
Edge computing is quickly growing because of its ability to support AI and ML and because of its inherent advantages. Its main benefits are:
- Reduced latency
- Real-time analytics
- Low bandwidth consumption
- Improved security
- Reduced costs
Edge AI systems capitalize on these advantages and can run machine learning algorithms on existing CPUs or even less capable microcontrollers (MCUs).
Compared to other applications that utilize AI chips, Edge AI delivers superior performance, especially regarding latency in data transfer and eliminating security threats in the network.
To learn more about what Edge AI is and how it works, read our blog What is Edge AI and Why Does it Matter?
Edge AI Unlocks Many Benefits for End Users
The ability to process AI on devices or close to the data source unlocks 11 impressive benefits for companies. These include:
- Improved bandwidth efficiency
- Reduced latency
- Fewer size and weight constraints
- Enhanced privacy
- Lower hardware costs
- The ability to function offline
- High availability
- Improved model accuracy
- Sustainability advantages
- Lower costs compared to cloud-based AI
- Increased levels of automation
There are also use cases that benefit tremendously from Edge AI. These include banking, content and ad services, delivery logistics systems, home security systems, weather technologies, recommendation engines, and more.
Edge AI helps make all of this possible, and as we continue to build ever-stronger technologies, it’s important to think about how they aid our quality of life.
To find out more, look at our blog 11 Impressive Benefits and Use Cases of Edge AI.
Understanding the Advantages and Disadvantages of Edge AI
Two technologies that impact data flow management are Edge computing and Edge AI.
The quantity of data generated by business transactions is growing exponentially. To help manage these extraordinary amounts of data, new and innovative methods of data flow management have made their way onto the playing field.
Edge computing is one of these methods as it analyzes and stores data closer to users. This data storage and analysis can take place on Edge-enabled devices, taking pressure off centralized servers by lessening the amount of data sent to them.
The combination of Edge computing and AI has created a new frontier known as Edge AI. Edge AI takes advantage of the many benefits of Edge Computing, such as reduced latency, improved bandwidth, and the ability to function offline.
Like all technologies, Edge computing and Edge AI have advantages and disadvantages. Users must consider this to determine if Edge technologies are a good fit for them and their business.
The advantages of Edge AI include:
1. Reduced latency
Edge AI takes some of that load off the cloud platform, reducing latency. Additionally, by analyzing locally, the return time from the cloud to the company using the information is reduced, leaving the cloud-based platform free for other tasks such as analytics.
2. Reduced bandwidth
Just as Edge AI offers reduced latency, it also reduces bandwidth. Because more data is processed, analyzed, and stored locally, less data is sent to the cloud. This reduction in data flow minimizes user costs.
3. Security
Edge AI results in less data being sent to centralized cloud storage. The situation of having “all your eggs in one basket” is minimized by processing and storing some data in an edge network. If data does need to go to the cloud, the Edge filters redundant, extraneous, and unneeded data, sending only what’s important.
4. Scalability and Versatility
As Edge devices become more common, they’re often available for cloud-based platforms. Additionally, as OEM equipment manufacturers make Edge capability native to their equipment, the system will be much easier to scale. This proliferation also allows local networks to remain functional even when upstream or downstream nodes are down.
The disadvantages of Edge AI include:
1. Lost data
When implemented, an Edge AI system must be thoroughly planned out and programmed to avoid data loss. Many Edge devices discard irrelevant data after collection (as they should), but if the data dumped is relevant, that data is lost, and any analysis will be flawed.
2. Security
Just as there’s a security advantage at the cloud and enterprise levels, there’s a security risk at the local level. It does a company no good to have a cloud-based provider with excellent security only to have their local network open to breach. While cloud-based security is becoming more robust, it’s usually human error and locally used applications and passwords that open the door to most breaches.
3. Less compute power
Edge AI is excellent, but it still lacks the computing power available for cloud-based AI.
Because of this, only select AI tasks can be performed on an Edge device. Cloud computing will still handle creating and serving large models, but Edge devices can perform on-device inference with smaller models. Edge devices can also handle small transfer learning tasks.
4. Machine variations
Relying on Edge devices means there’s significantly more variation in machine types. So, failures are more common.
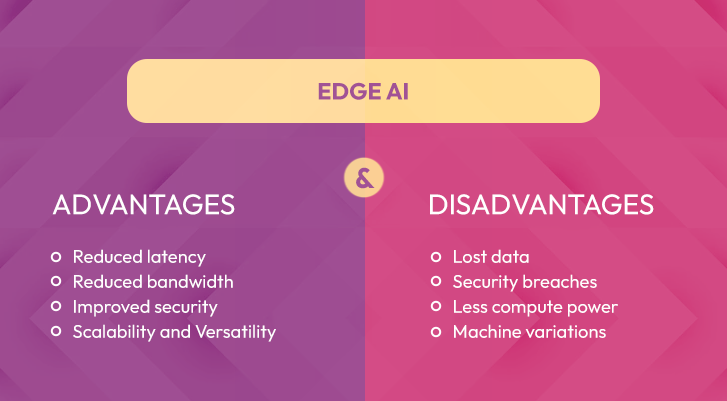
To find out more about the advantages and disadvantages of Edge AI, check out our blog The Advantages and Disadvantages of Edge AI Simply Explained.
Is Edge AI or Cloud AI Better for Security and Privacy?
While the cloud offers many benefits, it suffers significant privacy and security issues, making Edge-based AI an attractive option for many companies.
One big issue with Cloud-based AI is that it puts data out of users’ hands.
Instead of keeping sensitive data close by, cloud customers send their data to a large company with a centralized data center that is sometimes very far away.
This comes with privacy risks as cloud data centers are susceptible to hacking, not to mention that data is much more likely to be intercepted and tampered with when in transit.
Edge AI, on the other hand, provides incredible data security and privacy precisely because data is processed locally rather than within centralized servers.
While this isn’t to say that Edge-enabled devices are immune to security and privacy risks, it does mean that there’s less data being sent to and from a server, meaning that there’s a less complete collection of data that hackers can attack at any given time.
Another advantage is that Edge AI-enabled devices tend to hold minimal amounts of data. So, even in cyberattacks, hackers can only get their hands on a small amount of data and not complete data sets.
When cloud-based AI is in use, privacy can easily be compromised in an attack because cloud servers often contain complete information about locations, events, and people.
In contrast, because Edge AI only creates, processes, and analyzes the data required in a specific instance, other sensitive data can’t be tampered with in the event of an attack.
To find out more, see our blog Is the Edge or Cloud Better for Security and Privacy?
Edge AI is Booming – Here Are Its Top 5 Use Cases
Several AI use cases are best suited to the Edge, where processing occurs at or close to the data source, reducing latency, lowering costs, increasing data privacy, and improving reliability.
Thanks to the inherent benefits seen at the Edge, many companies are investing in Edge solutions, spurring innovation, and, of course, new Edge AI use cases.
5 of the top Edge AI use cases include:
- Security and surveillance
- The industrial IoT
- Autonomous vehicles
- Healthcare use cases
- Smart home use cases
These examples highlight how Edge AI is positively impacting the world today. They showcase some of the most significant innovations and ingenuity Edge AI can offer users at home and work.
For more information, check out our article Edge AI Brings Fascinating New Use Cases. We Cover the Top 5 Here.
Discover the Top 11 Industry Examples of Edge AI
Edge AI brings processing and storage capabilities closer to where they’re needed. As a result, Edge AI technologies can be used in various industries to great benefit.
The Top 11 Industry examples of Edge AI include:
1. Speech recognition
Speech recognition algorithms that transcribe speech on mobile devices.
2. Health monitors
Using local AI models, wearable health monitors assess heart rate, blood pressure, glucose levels, and breathing.
3. Robotic arms
Robot arms gradually learn better ways to grasp particular packages and then share this information with the cloud to improve other robots.
4. Item counting Edge AI at checkouts
Edge AI cashier-less services such as Amazon Go automatically counts items placed into a shopper’s bag without a separate checkout process.
5. Edge AI optimized traffic control
Smart traffic cameras that automatically adjust light timings to optimize traffic.
6. Autonomous truck convoys
Autonomous vehicles facilitate automated platooning of truck convoys, allowing drivers to be removed from all trucks except the one in front.
7. Remote asset monitoring
Remotely monitoring assets in the oil and gas industry where Edge AI enables real-time analytics with processing much closer to the asset.
8. Edge AI energy management
Smart grids, where Edge computing will be a core technology, can help enterprises manage their energy consumption better.
9. Fault detection in product lines
Edge AI helps manufacturers analyze and detect changes in their production lines before a failure occurs.
10. Patient monitoring
Edge AI patient monitoring processes data locally to maintain privacy while enabling timely notifications.
11. Self-driving cars
Autonomous vehicles can connect to the edge to improve safety, enhance efficiency, reduce accidents and decrease traffic congestion.
To explore these Edge AI use cases in more detail, see our blog Discover the Top 11 Edge AI Use Cases for Industries.
How Can Orchestrait Help Overcome the Challenges of Edge AI?
Edge AI is proven and here to stay, but, like all technology, it’s not without its challenges. Many factors make Edge AI difficult to implement.
Some of these include optimization problems, limited expertise and a lack of knowledge, and the time and costs of Edge AI development and deployment.
In addition, it’s also imperative for companies using Edge AI to keep up with advancements in the market.
Xailient’s Orchestrait solves optimization problems, addresses the issue of limited expertise, improves the time and costs associated with Edge AI deployment, and assists with maintenance (an important factor in the data management cycle).
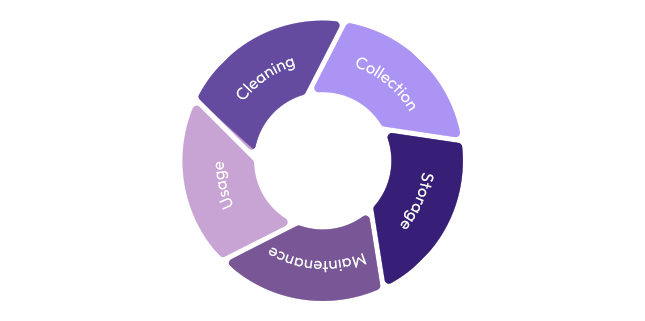
It achieves this by providing a platform that offers developers in the smart home space an easy, automatic, and cost-effective Edge AI maintenance solution.
To learn more about Orchestrait and how it remedies some of the biggest challenges of Edge AI, see our blog Edge AI Brings Challenges, but Xailient’s Orchestrait Has the Solution.
Edge AI is Transforming Enterprises
Edge AI can provide limitless scalability and incredible performance, enabling businesses to use their data efficiently. That’s why many global companies are already reaping the benefits of Edge AI.
Edge AI can significantly benefit numerous industries, from driving autonomous vehicles to improving production monitoring of assembly lines.
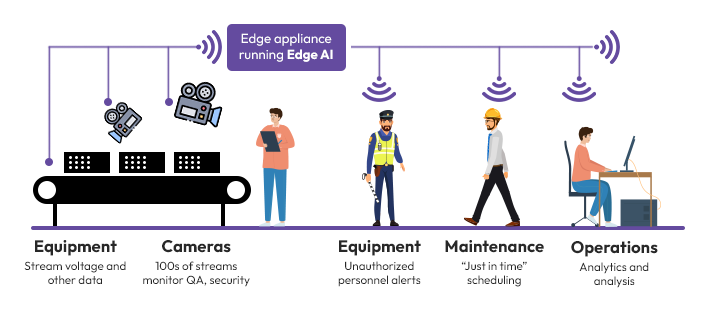
On top of this, the recent 5G roll-out in many countries offers Edge AI an extra boost as more industrial Edge AI applications continue to emerge.
Edge AI can benefit enterprises in the following ways:
- Edge AI is an efficient asset management tool
- Edge AI guarantees better customer satisfaction
- Edge AI aids large-scale infrastructure and Edge device life-cycle management
As can be seen, implementing Edge AI is a wise decision for many businesses.
To find out more, see our blog post Discover How Edge AI is Transforming Enterprises for the Better.
Edge AI Brings a Host of New Benefits to the HomeCam Space
Edge-based AI technologies have become increasingly available and affordable for computer vision applications. This allows the technology to make its way into everyday consumer products like HomeCams and other smart home products.
HomeCams backed by Edge AI offer both state-of-the-art security and convenience.
For example, imagine that a home security camera recognizes your face as you walk into your house and automatically adjusts the temperature, lights, and music to your preferred settings. If your housemate gets home first, the system can adjust to their favorite presets.
On the security front, when familiar faces are captured, these HomeCams will realize they are your family members or friends and won’t trigger alarms. Otherwise, they will send you instant alerts so that you know someone may intrude onto your property.
With strong Edge AI algorithms, Face Recognition HomeCams can greatly alleviate false alarms triggered by changing lighting conditions, moving trees, and the wind.
It gets better. HomeCams with Edge AI can be trained to detect packages, pets, and other objects in or outside the home.
Edge AI also makes it possible to recognize faces covered with sunglasses or a mask, which is almost impossible for conventional security cameras. They also offer drastically reduced latency compared to cloud-based HomeCam solutions.
To find out more, see our blog How does Edge AI Bring Benefits to the HomeCam Space?
Edge AI Brings a Diverse Array of Benefits to Video Analytics
Edge video analytics systems can execute computer vision and deep-learning algorithms through Edge AI chips embedded directly into cameras or with an attached Edge computing system.
Edge computing provides opportunities to move AI workloads from the Cloud to the Edge for video analytics, offering improved response times and bandwidth savings, among other advantages. For this reason, interest in Edge AI technologies is growing fast.
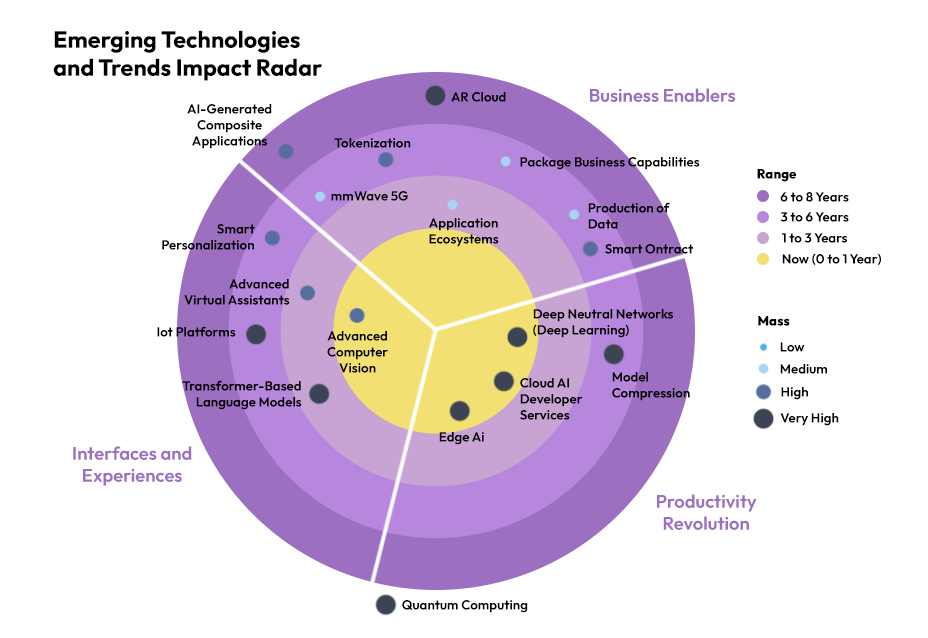
The benefits of Edge deployment are powerful when it comes to computer vision models that take large data streams as input like images or live video.
The enhanced processing efficiency of Edge AI technology has brought tangible improvements to AI-assisted video devices. This impacts the quantity and quality of achievable tasks thanks to the excellent bandwidth efficiency, improved latency, and reduced power constraints that Edge AI offers.
As Edge AI tools continue to improve and become more powerful, they’ll lead the way to a new era of intelligent video analytics with rapidly expanding capabilities and possible uses.
To learn more, check out our blog post What is Edge AI Video Analytics and Why it Matters.
Edge AI positively impacts the IoT
The IoT (internet of things) can be a powerful business asset. Still, the data generated by these devices has a long way to travel if a business solely relies on cloud computing and cloud-based AI.
Additionally, as companies continue to invest in IoT, adding sensors to legacy assets, their bandwidth load will quickly become heavy.
Edge intelligence addresses these issues by delivering real-time insights directly within operations where data is generated.
Most IoT configurations work like this: Sensors or devices are connected directly to the internet through a router, providing raw data to a backend server. On these servers, machine learning algorithms help predict various cases that might interest managers.
However, things get tricky when too many devices clog the network. For example, perhaps there’s too much traffic on local Wi-Fi or data being piped to the remote server. To help alleviate these issues, machine learning algorithms can be deployed on local servers or the devices themselves.
This is known as Edge AI.
Edge AI benefits the IoT by running machine learning algorithms on locally operated computers or embedded systems instead of on remote servers. It provides the opportunity to sort through data and decide what’s relevant before sending it off to a remote location for further analysis.
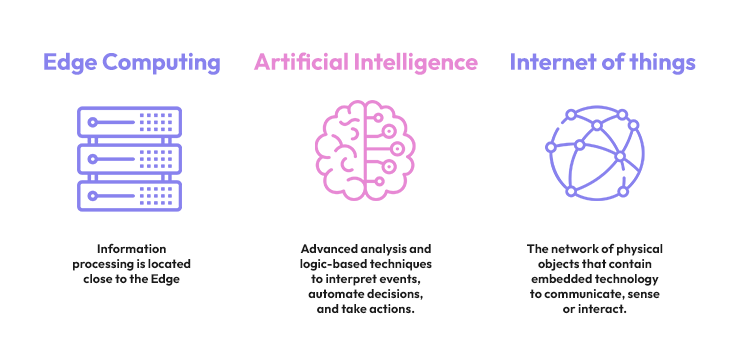
Additionally, Edge AI for the IoT improves performance as it can make decisions virtually without latency. This is possible because data analysis can be done entirely on an Edge-enabled IoT device.
If this topic takes your fancy, read our article How does Edge AI Boost the IoT’s Potential?
The Differences Between Edge AI and Cloud AI
The differences between Edge AI and Cloud AI come into play primarily for machine learning or deep learning use cases. This is the case because deep learning algorithms require intensive processing, making the performance of the hardware used a significant factor.
Cloud AI can provide good performance, but most deep learning applications can’t compromise on latency in the data transfer process, nor can they compromise on security threats in the network. For these reasons, Edge AI is an attractive solution for artificial intelligence applications.
As discussed earlier, power consumption is always a factor that intervenes in decisions about Edge AI processing. These concerns are understandable as heavy computations require a higher power supply.
The good news is that current Edge AI processors have AI accelerators with higher performance and low power consumption. While GPUs and TPUs still require high power, design and circuit architecture improvements will soon overpower this issue.
This is allowing more and more companies to deploy artificial intelligence (AI) and machine learning (ML) solutions at the Edge.
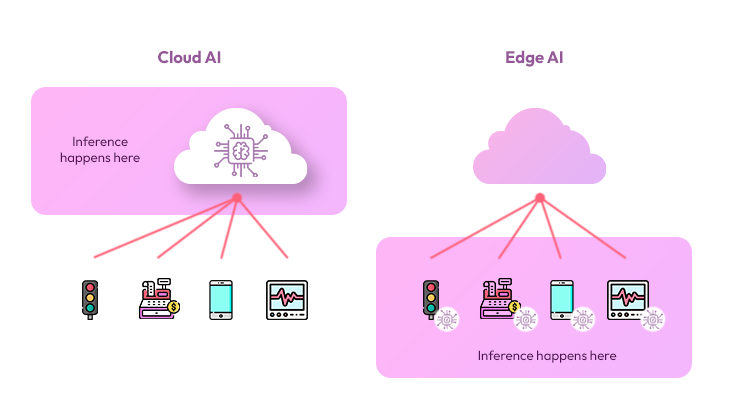
When AI and ML algorithms run locally on devices (at the edge of the network), Edge AI devices can process data, independently make decisions, offer virtually no latency, and generate valuable insights when internet connections are down.
Check out our blog Edge AI vs Cloud AI – Which One’s Better for Business? for more info.
Edge AI Chips Offer an Appealing Solution for the IoT
Edge computing has established itself as an attractive solution for IoT devices providing high-quality, actionable sensor data in a quick energy-efficient manner.
To fully realize this potential, researchers and industry leaders alike have been racing to develop new, specialized chips that can complete computationally demanding tasks on-device.
As a result of these efforts, the Edge AI chip has progressively become an appealing answer for AI applications and IoT gadgets.
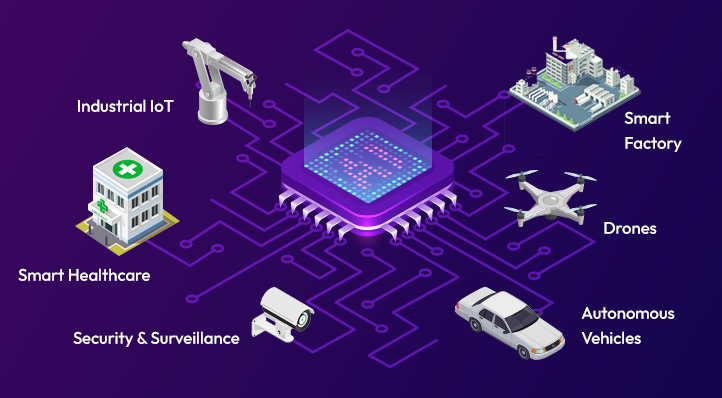
Established vendors and start-ups have jumped to complement or displace conventional controllers and microprocessors.
AI analytics at the Edge offers users a smooth experience without requiring data processing trips to cloud computing services. For the vendors, it has the potential to generate a lot of revenue.
As sensor data is increasingly handled at the Edge, Edge AI processing will likely spur overall IoT market growth, with Edge AI chips finding their way into an increasing number of consumer products such as smart home devices.
Edge AI chips will also enter multiple enterprise markets in the form of robots, cameras, sensors, and other IoT devices.
All in all, Edge computing has undeniably laid a good foundation for itself as an alluring answer for furnishing IoT gadgets with top-caliber sensor information in an investment-effective way.
This has left scientists and industry pioneers scrambling to foster new chips that can perform AI undertakings at the Edge.
To learn more about Edge AI chips and the benefits they offer the IoT, explore our blog What are Edge AI Chips and How do They Relate to the IoT?
Why Do We Need Edge AI Hardware?
With a growing demand for real-time deep learning workloads, specialized Edge AI hardware that allows for fast deep learning on-device has become increasingly necessary.
On top of this, today’s standard (cloud-based) AI approach isn’t enough to cover bandwidth, ensure data privacy, or offer low latency. Hence, AI tasks need to move to the Edge.
You might be wondering if 5G will solve these problems.
While 5G promises low latency, it will still need to rely on Edge computing to achieve its speeds in the lab. The 5G roll-out has been slow and doesn’t address bandwidth or privacy issues. Edge technologies are still highly relevant. This is exemplified by companies choosing to move their AI workloads to the Edge of the network.
As a result of this movement to the Edge, recent Edge AI trends are driving the need for specific AI hardware for on-device machine learning inference.
Specialized AI hardware, also called AI accelerators, accelerates data-intensive deep learning inference on Edge devices cost-effectively.
When it comes to computer vision, the workloads are high and incredibly data-intensive. Because of this, using AI hardware acceleration for Edge devices has many advantages. These include:
- better speed and performance
- greater scalability and reliability
- offline capabilities
- better data management
- improved privacy
While deep learning inference can be carried out in the cloud, the need for Edge AI is growing rapidly due to bandwidth, privacy concerns, and demand for real-time processing.
This makes AI hardware acceleration for Edge devices an appealing option for many computer vision tasks.
To learn more about Edge AI hardware, check out our article What is Edge AI hardware and why is it so important?
Deep Learning is Moving to the Edge to Benefit from Edge AI
Deep learning is a machine learning technique that teaches computers to do what comes naturally to humans: learn by example.
Deep learning models can achieve state-of-the-art accuracy. Sometimes they can even exceed human-level performance. Deep learning models are trained using a large set of data and neural network architectures containing many layers.
Just about any problem that requires “thought” is a problem deep learning can learn to solve.
Depending on the target application of a deep learning model, it may require low latency, enhanced security, or long-term cost-effectiveness.
Hosting deep learning models on the Edge instead of the cloud can be the best solution in such cases. This is because Edge deep learning runs deep learning processes on the individual device or an environment closer to the device than the cloud. This reduces latency, cuts down on high cloud processing costs, and improves security.
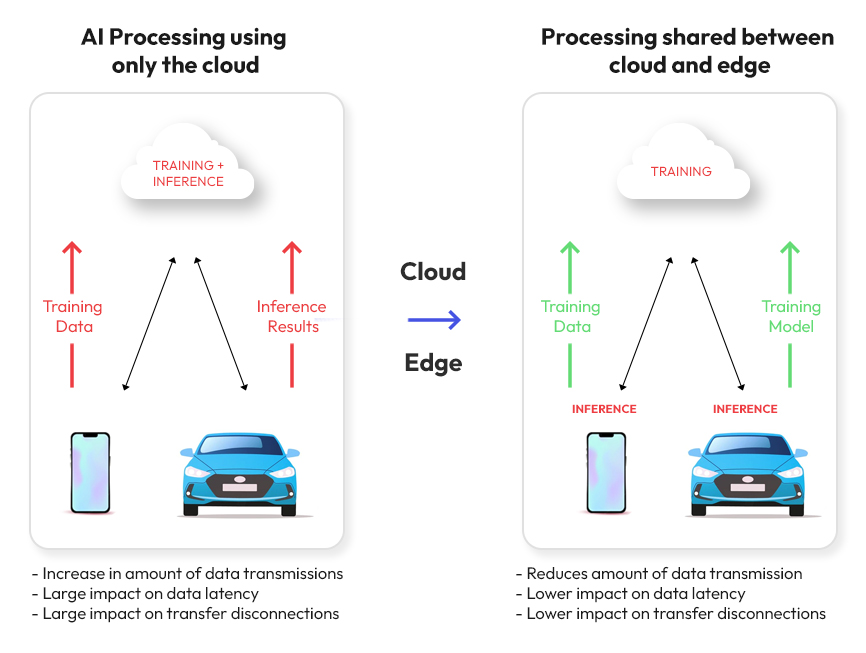
Despite this, there is still uncertainty in choosing between Edge AI and Cloud AI for deep learning use cases. As deep learning algorithms require intensive processing, the performance of the hardware becomes a significant factor.
Cloud AI can provide excellent performance for the system, but most deep learning applications cannot compromise with latency in data transfer and security threats in a network. This makes Edge AI an attractive option for artificial intelligence applications compared to cloud-based solutions.
As cloud alone isn’t necessarily a fantastic option for AI applications, a hybrid of Edge and Cloud AI can provide better performance.
For example, as trained models need to be updated for real-time data, this training can be done in the cloud, but the real-time data can be processed on the hardware through Edge AI for generating output.
In this instance, the processing division brings out the best of both technologies. Thus, it provides a better option than cloud-based AI on its own. However, it’s still important to remember that most applications need quicker real-time updated training, so Edge AI is often preferable to Cloud AI technology.
To learn more, visit our blog post How does Edge AI benefit deep learning?
How can Synthetic Data improve Edge AI data Collection and Training?
Our society largely depends on technology like artificial intelligence, and this is only going to increase in the future.
As we prepare for a world of incredible innovation, one thing is clear – data scientists and engineers are inherently limited by traditional methods of training Edge AI algorithms.
AI models can’t utilize any old dataset as ethical considerations and bias must be taken into account when training fair and effective Edge AI.
Experts and professionals in the field propose synthetic data as a revolutionary solution to ethical problems with data collection and training.
Synthetic Data refers to datasets that are artificially generated.
To create synthetic datasets, data scientists use randomly generated synthetic data to mask sensitive information while retaining statistically relevant characteristics from the original data.
Synthetic data can deliver perfectly labeled, realistic datasets and simulated environments at scale, allowing enterprises to overcome typical entry barriers to the AI market.
As a result, it’s predicted that synthetic data will ease the complex landscape of accelerated time-to-market schedules as it removes the need for companies to acquire large volumes of data which can be time-consuming and expensive.
To learn more about synthetic data and how it’s changing the Edge AI landscape, see our blog post What is Synthetic Data and How is it Creating Better Edge AI?
What is TinyML, and why is it important?
Machine learning (ML) is a type of AI that allows software applications to become more accurate at predicting outcomes without being explicitly programmed to do so. Machine learning algorithms use historical data as input to predict new output values.
ML uses data and algorithms to imitate how humans think and learn.
Recommendation engines are an everyday use case for machine learning, while other popular uses include fraud detection, spam filtering, malware threat detection, business process automation (BPA), and predictive maintenance.
TinyML, on the other hand, is the art and science of producing machine learning models frugal enough to work at the edge of a network. It’s a fast-growing field of machine learning technologies and applications, including hardware, algorithms, and software.
Whether it’s stand-alone IoT sensors, devices of many kinds, or autonomous vehicles, there’s one thing in common: Increasingly, data generated at the Edge is used to feed applications powered by machine learning models.
Machine learning models were never designed to be deployed at the Edge, but TinyML makes this possible.
TinyML can perform on-device sensor data analytics at extremely low power, enabling a variety of always-on use-cases and targeting battery-operated devices.
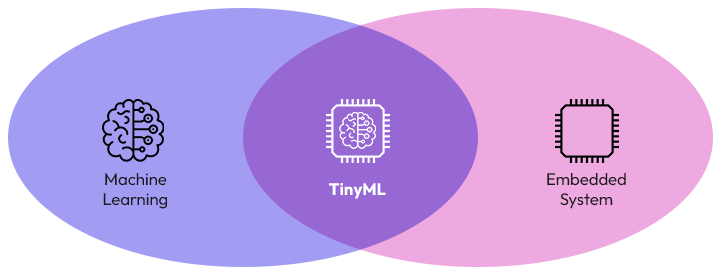
Analyzing large amounts of data based on complex machine learning algorithms requires significant computational capabilities. Therefore, data processing traditionally occurs in on-premises data centers or cloud-based infrastructure.
However, with the arrival of powerful, low-energy consumption IoT devices, computations can now be executed on Edge devices. This has given rise to the era of deploying advanced machine learning methods such as convolutional neural networks (CNNs) at the edges of the network.
How is Edge AI becoming the future of AI?
Due to the massive increase in data we are experiencing today, Edge computing and Edge AI are on their way to becoming indispensable technologies due to their ability to move data away from overburdened cloud data centers.
While the predicted global market capitalization of Edge computing infrastructure is estimated to be worth more than $800 billion by 2028, enterprises are investing heavily in artificial intelligence (AI).
While many companies are making Edge-related tech investments as a part of their digital transformation journey, forward-looking organizations and cloud companies see new opportunities by fusing Edge computing and AI (Edge AI), making Edge AI part of the future of computing on the Edge.
Edge AI will transform enterprises because the models have an optimized infrastructure for Edge computing that can handle bulkier AI workloads on the Edge and near the Edge.
Because of this, Edge AI can provide industry-leading performance and limitless scalability that enables businesses to use their data efficiently.
To learn more, read our blog Edge Computing and Edge AI: What to Expect in the Future
The Market for Edge AI Technologies is Set to Boom
According to a report published by MarketsandMarkets Research, the global Edge AI software market is set to grow from USD 590 million in 2020 to USD 1.83 trillion by 2026.
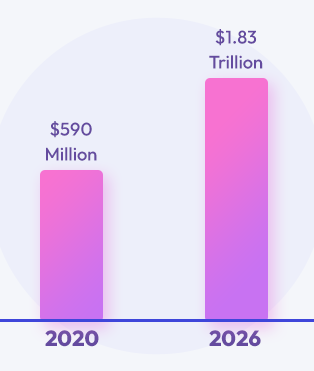
Various factors such as increasing enterprise workloads on the cloud and rapid growth in the number of intelligent applications are expected to drive the adoption of Edge AI solutions and services.
Edge AI software vendors have implemented various organic and inorganic growth strategies, such as new product launches, product upgrades, partnerships and agreements, business expansions, and mergers and acquisitions to strengthen their offerings in the market.
Major vendors in the global Edge AI software market include, from the US:
- Alphabet Inc.
- Microsoft Corporation
- IBM Corporation
- Amazon Web Services, Inc.
- Nutanix
- Synaptics Incorporated
- SWIM.AI Inc.
- TIBCO Software Inc.
- FogHorn Systems
- Azion Technologies LLC
- ClearBlade Inc.
- Alef Edge Inc.
- Adapdix
- Adapdix
- Reality Analytics Inc.
- edgeworx
- Kneron Inc.
- Veea Inc.
- Tact.ai Technologies, Inc.
from Europe:
- Imagimob AB (Sweden)
- Octonion SA. (Switzerland)
- Bragi (Germany)
- SixSq Sàrl (Switzerland)
- byteLAKE (Poland)
from Asia:
- Gorilla Technology Group (Taiwan)
- Horizon Robotics (China)
from the Middle East:
- Anagog Ltd. (Israel)
- Deci (Israel)
from India:
- DeepBrainz AI
- StrataHive
from Canada:
- Invision AI Inc.
Check out our blog, The Edge AI Market – What’s Happening Now and in the Future? to find out more.
Edge Security is Helping Secure Devices that Use Edge AI
It’s no secret that Edge computing and Edge AI technologies are growing fast. But unfortunately, because of this, security concerns have also drastically increased.
Edge computing and Edge AI are on their way to becoming indispensable technologies due to their ability to move data away from overburdened cloud data centers. Both are relatively new technologies, and, unfortunately, cyber security is a divisive topic at the best of times, let alone when it comes to new problems in relatively uncharted waters.
One thing for sure is that the need for Edge security is growing because of the exponential rise in Edge technology that’s currently taking place.
Edge security is aimed at protecting data that lives or travels through devices. It also protects users’ sensitive data at the farthest reaches or the ‘edge’ of a company’s network.
The primary drivers of Edge computing and Edge security are mobile and IoT applications.
Mobile and IoT applications are exploding, creating the need for highly accessible, low-latency, high-performing, secure, and easily scalable platforms capable of processing the enormous amount of data generated and consumed at the Edge.
Edge AI and Edge computing have advantages over cloud computing and cloud-based AI in these areas. On top of this, additional measures can be taken to boost the security of Edge technologies. These security measures include:
- Creating a secure perimeter: Securing access to Edge computing resources through encrypted tunnels, firewalls, and access control.
- Securing applications: Ensuring that Edge computing devices run apps that can be secured beyond the network layer.
- Enabling early threat detection: Making sure providers implement proactive threat detection technologies to identify a potential breach as early as possible.
- Creating automated patch cycles: Ensuring that automated patching is in place to keep devices updated while reducing the risk of potential surface attacks.
- Managing vulnerabilities: Implementing measures to ensure the continued maintenance and discovery of known and unknown vulnerabilities.
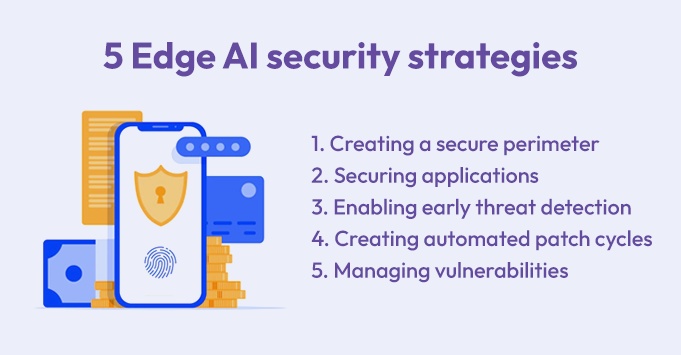
To learn about Edge security in more detail, have a look at our article, How is Edge Security Helping Secure Devices that Use Edge AI?
The final wrap on Edge AI
Thanks to recent innovations, Edge computing has paved the way for new AI opportunities that were previously unimaginable.
Today, almost all businesses have job functions that stand to benefit from the adoption of Edge AI. In addition, home users also reap the benefits of Edge AI technologies becoming more commonplace. Perhaps the most obvious example of this can be seen regarding smart home technologies.
Overall, this article has explored many components of Edge AI today and new opportunities that Edge AI technologies will enable in the future.
From explaining Edge AI to discussing its interactions with HomeCam technologies, video analytics, IoT, chips, hardware, deep learning and machine learning, security technologies, and cloud technologies, this article is an excellent guide to your most pressing Edge AI questions.
Glossary of Edge AI Terms
5G
5G is the fifth generation of mobile networks and wireless technology.
Following 4G, 5G will provide better network speeds for the ever-growing smartphone industry, enhance capabilities for calls and texts, and connect everyone everywhere across the globe.
5G has fewer loading time delays, is faster, and has more data capacity than any other generation. It’s the reliable upgrade from 4G that will improve many industries, such as agriculture, healthcare, and logistics.
AI
Artificial intelligence (AI) refers to machines or systems that mimic human intelligence to perform tasks and iteratively improve based on collected information.
AI systems work by taking in large amounts of labeled training data, analyzing the data for patterns and correlations, and using those findings to make predictions about future states.
AI significantly enhances human capabilities and contributions, making it a valuable business asset.
AI accelerators
AI accelerator is a term used to refer to high-performance parallel computation machines that are specifically designed for the efficient processing of AI workloads.
These computation machines make platforms significantly faster across a variety of models, applications, and use-cases.
AI accelerators can be grouped into three main categories: AI-accelerated GPU, AI-accelerated CPU, and dedicated hardware AI accelerators.
AI chips
Artificial intelligence (AI) chips include field-programmable gate arrays (FPGAs), graphics processing units (GPUs), and application-specific integrated circuits (ASICs), specialized for AI.
General-purpose chips like CPUs can be used for simple AI tasks, but they’re becoming less and less useful as AI becomes more sophisticated.
AI chips are speedy and efficient as they can complete multiple computations per unit of energy consumed. They achieve this by incorporating huge numbers of tiny transistors, which run faster and consume less energy than larger transistors.
Bandwidth
Bandwidth refers to the volume of information that can be sent over a connection in a measurable amount of time.
Usually measured in megabits, kilobits, or bits, bandwidth is a measure of throughput (amount per second) rather than speed (distance covered per second).
Gaming, streaming, running AI, and other high-capacity activities demand a certain amount of bandwidth speed to get the best user experience without any lag.
Bandwidth efficiency
Bandwidth efficiency is a term for the information rate transmitted over a given bandwidth in a communication system.
A high bandwidth network delivers more information than a low bandwidth network in the same amount of time.
As this makes the network feel faster, high bandwidth networks and connections are often referred to as “high-speed.”
Cloud
The term cloud refers to servers that are accessed over the internet. It also refers to the databases, software, and AI that run on those servers.
Cloud servers are located in data centers all over the world. When a device uses cloud computing, data must travel to these centralized data centers for processing before returning back to the device with a decision or action.
By implementing cloud computing, users don’t have to manage physical servers themselves or run software applications and AI on their own machines.
Cloud AI
Cloud AI combines artificial intelligence (AI) with cloud computing.
Cloud AI consists of a shared infrastructure for AI use cases, supporting numerous AI workloads and projects simultaneously.
Cloud AI brings together AI, software, and hardware (including open source), to deliver AI software-as-a-service.
It facilitates enterprises’ access to AI, enabling them to harness AI capabilities.
Cloud computing
Cloud computing refers to the delivery of different services through the internet.
These resources include applications and tools like data storage, databases, servers, and software.
Rather than storing files, running applications, and generating insights on a local Edge device or Edge server, cloud-based solutions take care of these tasks in a remote database.
As long as an electronic device has access to the web, it has access to the data and the software programs offered by the cloud.
CNN
A convolutional neural network (CNN) is a type of artificial neural network used in image processing and recognition that’s specially designed to process pixel data.
A CNN is a powerful image processing AI that uses deep learning to perform both descriptive and generative tasks. It typically uses machine vision that includes video and image recognition technologies.
Computer vision
Computer vision is a field of artificial intelligence (AI) that enables systems and computers to obtain meaningful information from videos, digital images, and other visual inputs — and make recommendations or take actions based on that information.
CPU
A Central Processing Unit (CPU) refers to the primary component of a computer that processes instructions.
The CPU runs operating systems and applications, constantly receiving input from active software programs or the user.
It processes data and produces outputs, which may be displayed on-screen or stored by the application. CPUs contain at least one processor, which is the actual chip inside the CPU that executes calculations.
CVOps
Cameras that look out a front door are doing a different job to the cameras looking down on a backyard from the roofline. Both watch for people to keep users safe, but in the world of AI these are very different tasks.
CVOps is a category that describes the enterprise software process for delivering the right Computer Vision to the right camera at the right time.
A reliable, accurate CV system needs to collect data over time (to deal with Data Drift and Model Drift), and to deliver software updates that adapt to the changing physical world.
Edge
The term ‘Edge’ in Edge computing refers to where computing and storage resources are located.
The ‘Edge’ refers to the end points of a network such as user devices. Edge computing keeps compute and storage at the same point (or as close as possible to) where data is initially generated.
Edge AI
Edge artificial intelligence (Edge AI) combines Artificial Intelligence and Edge computing.
With Edge AI, algorithms are processed locally, either directly on user devices or servers near the device.
The AI algorithms use data generated by these devices, allowing them to make independent decisions in milliseconds without connecting to the cloud or the internet.
Edge AI algorithms
Edge AI algorithms process data generated by hardware devices at the local level, either directly on the device or on the nearby server.
Edge AI algorithms utilize data that’s generated at the local level to make decisions in real time.
Edge AI hardware
Edge AI hardware refers to the many devices that are utilized to power & process artificial intelligence at the Edge.
These gadgets are at the Edge because they have the capacity to process artificial intelligence on the hardware itself, rather than relying on sending data to the cloud.
Edge computing
Edge computing is a distributed computing method that keeps data processing and analysis closer to data sources such as IoT devices or local Edge servers.
Keeping data close to its source can deliver substantial benefits, including lower costs, improved response times, and better bandwidth availability.
Edge devices
An Edge device is a piece of hardware that controls data flow at the boundary between two networks.
Edge devices fulfill a variety of roles, but they all serve as network exit or entry points.
Some common Edge device functions include the routing, transmission, monitoring, processing, storage, filtering, and translation of data passing between networks.
Edge devices can include an employee’s notebook computer, smartphones, various IoT sensors, security cameras, or even the internet-connected microwave in an office lunchroom.
Edge network
An Edge network refers to the area where a local network or device teams up with the internet.
This area is geographically close to the devices it’s communicating with and can be thought of as an entry point to the network.
Embedded Edge AI
Embedded Edge AI refers to Edge AI technology that’s built-in or embedded within a device. This is done by incorporating specialized Edge AI chips into products.
GPU
A GPU, or a graphics processing unit, is a specialized processor.
These processors can manipulate and alter memory to accelerate graphics rendering.
GPUs can process many pieces of data simultaneously, making them useful for video editing, gaming, and machine learning use cases. They’re typically used in embedded systems, personal computers, smartphones, and gaming consoles.
HomeCams
HomeCams are a type of home security camera that can function indoors or outdoors, picking up faces and identifying who people are inside and around a property.
When HomeCams are installed, they act as a defender of your home, sending instant alerts when people are detected.
IoT
The term internet of things (IoT) refers to the collective network of connected devices and the technology that allows communication between devices and the cloud, and between the devices themselves.
Thanks to the rise of high bandwidth telecommunications and cost-effective computer chips, billions of devices are connected to the internet.
In fact, everyday devices like vacuums, toothbrushes, cars, and other machines can use sensors to collect data and respond intelligently to users, making them part of the IoT.
IoT applications
IoT applications are defined as a collection of software and services that integrate data received from IoT devices.
They use AI or machine learning to analyze data and make informed decisions.
IoT devices
IoT devices are hardware devices, such as appliances, gadgets, sensors, and other machines that collect and exchange data over the Internet.
Different IoT devices have different functions, but they all work similarly.
IoT devices are physical objects that sense things going on in the physical world. They contain integrated network adapters, firmware, CUPs, and are usually connected to a Dynamic Host Configuration Protocol (DHCP) server.
Latency
Latency is another word for delay.
When it comes to IT, low latency is associated with a positive user experience while high latency is associated with a poor one.
In computer networking, latency is an expression of how much time it takes for data to travel from one designated point to another. Ideally, latency should be as close to zero as possible.
Depending on the application, even a relatively small increase in latency can ruin user experiences and render applications unusable.
Machine learning
Machine learning (ML) is a type of artificial intelligence (AI).
Machine learning allows software applications to become more accurate at predicting outcomes without explicitly being programmed to perform such tasks.
Machine learning algorithms predict new output values using historical data as input.
Machine learning algorithms
A machine learning algorithm is a method AI systems can implement to conduct their tasks. These algorithms generally predict output values from given input data.
Machine learning algorithms perform two key tasks – classification and regression.
Microcontrollers
A microcontroller, sometimes referred to as an embedded controller or a microcontroller unit (MCU), is a compact integrated circuit.
Microcontrollers are designed to govern specific operations in embedded systems. The usual microcontroller includes a processor, memory, and input/output peripherals, all contained on the one chip.
Microcontrollers can be found in many devices and are essentially simple, miniature personal computers designed to control small features of a large component without a complex front-end operating system.
Microprocessors
Microprocessors are computer processors where data processing control is included on one or more integrated circuits.
Microprocessors can perform functions similar to a computer’s central processing unit.
Orchestrait
Orchestrait is an AI management platform that automates and ensures Face Recognition data privacy compliance across all jurisdictions.
Orchestrait allows companies to monitor the performance of AI algorithms on all IoT devices, allowing them to continuously improve their Edge AI models no matter how large the fleet.
TinyML
Tiny machine learning (TinyML) is a fast-growing field involving applications and technologies like algorithms, hardware, and software capable of performing on-device sensor data analytics at low power.
TinyML enables numerous always-on use cases and is well-suited to devices that use batteries.
TPU
Tensor Processing Units (TPUs) are hardware accelerators for machine learning workloads.
They were designed by Google and came into use in 2015.
In 2018, they became available for third-party use as part of Google’s cloud infrastructure. Google also offers a version of the chip for sale.
Video analytics
Video analytics is a type of AI technology that automatically analyzes video content to carry out tasks such as identifying moving objects, reading vehicle license plates, and performing Face Recognition.
While popular in security use cases, video analytics can also be used for quality control and traffic monitoring, among many other important use cases.
Wearables
The term wearables refers to electronic technology or devices worn on the body. Wearable devices can track information in real time.
Two common types of wearables include smartglasses and smartwatches.